By Emily Saltz, Tommy Shane, Victoria Kwan, Claire Leibowicz, Claire Wardle. This post has been republished with permission by the Partnership on AI. The original post was published on Medium.
Manipulated photos and videos flood our fragmented, polluted, and increasingly automated information ecosystem, from a synthetically generated “deepfake,” to the far more common problem of older images resurfacing and being shared with a different context.
While research is still limited, there is some empirical support to show visuals tend to be both more memorable and more widely shared than text-only posts, heightening their potential to cause real-world harm, at scale. Consider the numerous audiovisual examples in this running list of hoaxes and misleading posts about police brutality protests in the United States. In response, what should social media platforms do? Twitter, Facebook, and others have been working on new ways to identify and label manipulated media.
Label designs aren’t applied in a vacuum, as the divisive reactions to Twitter’s recent decision to label two of Trump’s misleading and incendiary tweets demonstrate. In one instance, the platform appended a label under a tweet that contained incorrect information about California’s absentee ballot process, encouraging readers to click through and “Get the facts about mail-in ballots.” In the other instance, Twitter replaced the text of a tweet referring to shooting of looters with a label that says: “This tweet violates the Twitter Rules about glorifying violence.” Twitter determined that it was in the public interest for the tweet to remain accessible and this label allowed users the option to click through to the original text.
These examples show that the world notices and reacts to the way platforms label content, including President Trump himself, who has directly responded to the labels. With every labeling decision, the ‘fourth wall’ of platform neutrality is breaking down. Behind it, we can see that every label — whether for text, images, video, or a combination — comes with a set of assumptions that must be independently tested against clear goals and transparently communicated to users. Each platform uses its own terminology, visual language, and interaction design for labels, with application informed respectively by their own detection technologies, internal testing, and theories of harm (sometimes explicit, sometimes ad hoc). The end result is a largely incoherent landscape of labels leading to unknown societal effects.
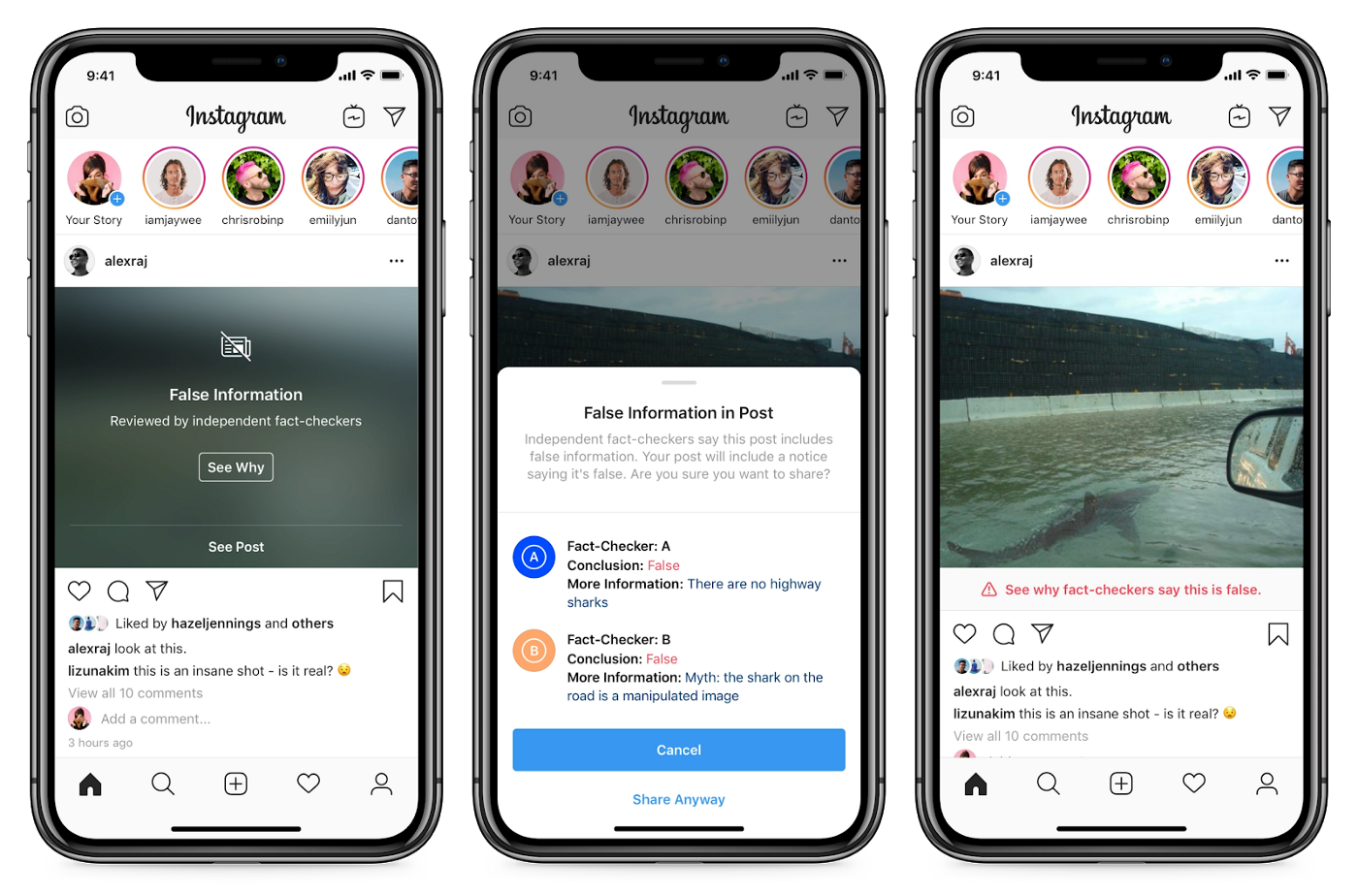
For an example of labeling manipulated media, see Facebook’s Blog: Combatting Misinformation on Instagram
At the Partnership on AI (PAI) and First Draft, we’ve been collaboratively studying how digital platforms might address manipulated media with empirically-tested and responsible design solutions. Though definitions of “manipulated media” vary, we define it as any image or video with content or context edited along the “cheap fake” to “deepfake” spectrum with the potential to mislead and cause harm.
In particular, we’re interrogating the potential risks and benefits of labels: language and visual indicators that notify users about manipulation. What are best practices for digital platforms applying (or not applying) labels to manipulated media to reduce mis- and disinformation’s harms?
What we’ve found
To reduce mis/disinformation’s harm to society, we have compiled the following set of principles for designers at platforms to consider for labeling manipulated media. We also include design ideas for how platforms might explore, test, and adapt these principles for their own contexts. These principles and ideas draw from hundreds of studies and many interviews with industry experts, as well as our own ongoing user-centric research at PAI and First Draft.
Ultimately, labeling is just one way of addressing mis/disinformation: how does labeling compare to other approaches, such as removing or downranking content? Platform interventions are so new that there isn’t yet robust public data on their effects. But researchers and designers have been studying topics of trust, credibility, information design, media perception, and effects for decades, providing a rich starting point of human-centric design principles for manipulated media interventions.
Principles
1. Don’t attract unnecessary attention to the mis/disinformation
Although assessing harm from visual mis/disinformation can be difficult, there are cases where the severity or likelihood of harm is so great — for example, when the manipulated media poses a threat to someone’s physical safety — that it warrants an intervention stronger than a label. Research shows that even brief exposure has a “continued influence effect” where the memory trace of the initial post cannot be unremembered [1]. With images and video especially sticky in memory due to “the picture superiority effect” [2] the best tactic for reducing belief in such instances of manipulated media may be outright removal or downranking.
Another way of reducing exposure may be the addition of an overlay. This approach is not without risk, however: the extra interaction to remove the overlay should not be framed in a way that creates a “curiosity gap” for the user, making it tempting to click to reveal the content. Visual treatments may want to make the posts less noticeable, interesting, and visually salient compared to other content — for example, using grayscale, or reducing the size of the content.
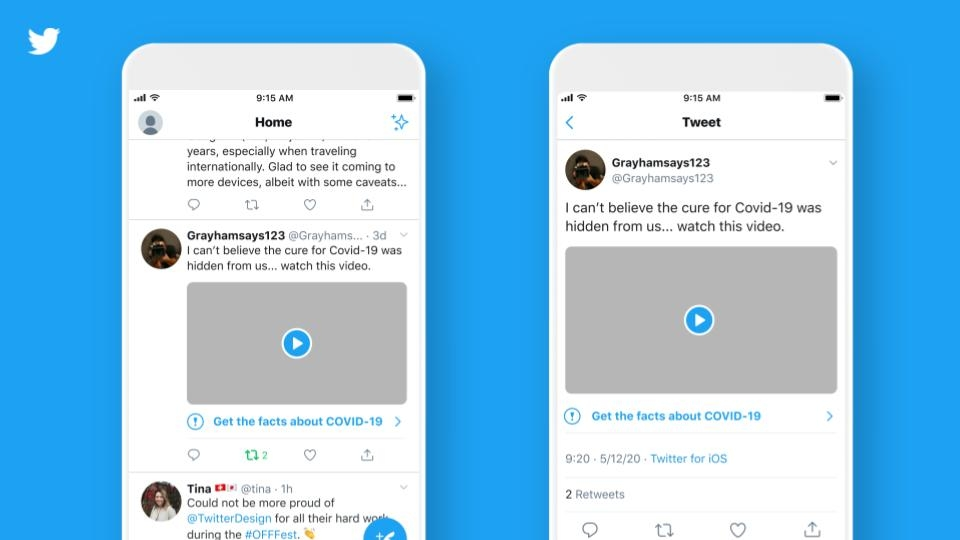
For an example of a manipulated media overlay, see Twitter’s Blog: Updating our Approach to Misleading Information
2. Make labels noticeable and easy to process
A label can only be effective if it’s noticed in the first place. How well a fact-check works depends on attention and timing: debunks are most effective when noticed simultaneously with the mis/disinformation, and are much less effective if noticed or displayed after exposure. Applying this lesson, labels need to be as or more noticeable than the manipulated media, so as to inform the user’s initial gut reaction [1], [2], [3], [4], [5].
From a design perspective, this means starting with accessible graphics and language. An example of this is how The Guardian prominently highlights the age of old articles with labels.
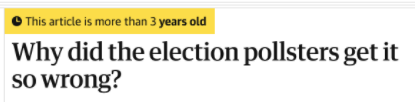
Articles on The Guardian signal when the story is dated: Why we’re making the age of our journalism clearer at the Guardian
The risk of label prominence, however, is that it could draw the user’s attention to misinformation that they may not have noticed otherwise. One potential way to mitigate that risk would be to highlight the label through color or animation, for example, only if the user dwells on or interacts with the content, indicating interest. Indeed, Facebook appears to have embraced this approach by animating their context button only if a user dwells on a post.
Another opportunity for making labels easy to process might be to minimize other cues in contrast, such as social media reactions and endorsement. Research has found that exposure to social engagement metrics increases vulnerability to misinformation [6]. In minimizing other cues, platforms may be able to nudge users to focus on the critical task at hand: accurately assessing the credibility of the media.
3. Encourage emotional deliberation and skepticism
In addition to being noticeable and easily understood, a label should encourage a user to evaluate the media at hand. Multiple studies have shown that the more one can be critically and skeptically engaged in assessing content, the more accurately one can judge the information [1], [2], [5], [7]. In general, research indicates people are more likely to trust misleading media due to “lack of reasoning, rather than motivated reasoning” [8]. Thus, deliberately nudging people to engage in reasoning may actually increase accurate recollection of claims [1].
One tactic to encourage deliberation, already employed by platforms like Facebook, Instagram, and Twitter, requires users to engage in an extra interaction like a click before they can see the visual misinformation. This additional friction builds in time for reflection and may help the user to shift into a more skeptical mindset, especially if used alongside prompts that prepare the user to critically assess the information before viewing content [1], [2]. For example, labels could ask people “Is the post written in a style that I expect from a professional news organization?” before reading the content [9].
4. Offer flexible access to more information
During deliberation, different people may have different questions about the media. Access to these additional details should be provided, without compromising the readability of the initial label. Platforms should consider how a label can progressively disclose more detail as the user interacts with it, enabling users to follow flexible analysis paths according to their own lines of critical consideration [10].
What kind of detail might people want? This question warrants further design exploration and testing. Some possible details may include the capture and edit trail of media; information on a source and its activity; and general information on media manipulation tactics, ratings, and mis/disinformation. Interventions like Facebook’s context button can provide this information through multiple tabs or links to pages with additional details.
5. Use a consistent labeling system across contexts
On platforms it is crucial to consider not just the effects of a label for a particular piece of content, but across all media encountered — labeled and unlabeled.
Recent research suggests that labeling only a subset of fact-checkable content on social media may do more harm than good by increasing users’ beliefs in the accuracy of unlabeled claims. This is because a lack of a label may imply accuracy in cases where the content may be false but not labeled, known as “the implied truth effect” [11]. The implied truth effect is perhaps the most profound challenge for labeling as it is impossible for fact-checkers to check all media posted to platforms. Because of this limitation in fact-checking at scale, fact-checked media on platforms will always be a subset, and labeling that subset will always have the potential to boost the perceived credibility of all other unchecked media, regardless of its accuracy.
Platform designs should, therefore, aim to minimize this “implied truth effect” at a cross-platform, ecosystem level. This demands much more exploration and testing across platforms. For example, what might be the effects of labeling all media as “unchecked” by default?
Further, consistent systems of language and iconography across platforms enable users to form a mental model of media that they can extend to accurately judge media across contexts, for example, by understanding that a “manipulated” label means the same thing whether it’s on YouTube, Facebook, or Twitter. Additionally, if a design language is consistent across platforms, it may help users more rapidly recognize issues over time as it becomes more familiar, and thus and easier to process and trust (see principle two, above).
6. Repeat the facts, not the falsehoods
It’s important not just to debunk what is false, but to ensure that users come away with a clear understanding of what is accurate. It’s risky to display labels that describe media in terms of the false information without emphasizing what is true. Familiar things seem more true, meaning every repetition of the mis/disinformation claim associated with a visual risks imprinting that false claim deeper in memory — a phenomenon known as “the illusory truth effect” or the “myth-familiarity boost” [1].
Rather than frame the label in terms of what is inaccurate, platforms should elevate the accurate details that are known. Describe the accurate facts rather than simply rate the media in unspecific terms while avoiding repeating the falsehoods [1], [2], [3]. If it’s possible, surface clarifying metadata, such as the accurate location, age, and subject matter of a visual.
7. Use non-confrontational, empathetic language
The language on a manipulated media label should be non-confrontational, so as not to challenge an individual’s identity, intelligence, or worldview. Though the research is mixed, some studies have found that in rare cases, confrontational language risks triggering the “backfire effect,” where an identity-challenging fact-check may further entrench belief in a false claim [13], [14]. Regardless, it helps to adapt and translate the label to the person. As much as possible, meet people where they are by framing the correction in a way that is consistent with the users’ worldview. Highlighting accurate aspects of the media that are consistent with their preexisting opinions and cultural context may make the label easier to process [1], [12].
Design-wise, this has implications for the language of the label and label description. While the specific language should be tested and refined with audiences, language choice does matter. A 2019 study found that when adding a tag to false headlines on social media, the more descriptive “Rated False” tag was more effective at reducing belief in the headline’s accuracy than a tag that said “Disputed” [15].
Additionally, there is an opportunity to build trust by using adaptive language and terminology consistent with other sources and framings of an issue that a user follows and trusts. For example, “one study found that Republicans were far more likely to accept an otherwise identical charge as a ‘carbon offset’ than as a ‘tax,’ whereas the wording has little effect on Democrats or Independents (whose values are not challenged by the word ‘tax;’ Hardisty, Johnson, & Weber, 2010)” [1].
8. Emphasize credible refutation sources that the user trusts
The effectiveness of a label to influence user beliefs may depend on the source of the correction. To be most effective, a correction should be told by people or groups the user knows and trusts [1], [16], rather than a central authority or authorities that the user may distrust. Research indicates that when it comes to the source of a fact-check, people value trustworthiness over expertise — that means a favorite celebrity or YouTube personality might be a more resonant messenger than an unfamiliar fact-checker with deep expertise.
If a platform has access to correction information from multiple sources, the platform should highlight sources the user trusts, for example promoting sources that the user has seen and/or interacted with before. Additionally, platforms could highlight related, accurate articles from publishers the user follows and interacts with, or promote comments from friends that are consistent with the fact-check. This strategy may have the additional benefit of minimizing potential retaliation threats to publishers and fact-checkers — ie preventing users from personally harassing sources they distrust.
9. Be transparent about the limitations of the label and provide a way to contest it
Given the difficulty of ratings and displaying labels at scale for highly subjective, contextualized user-generated content, a user may have reasonable disagreements with a label’s conclusion about a post. For example, Dr. Safiya Noble, a professor of Information Studies at UCLA, and author of the book “Algorithms of Oppression,” recently shared a Black Lives Matter-related post on Instagram that she felt was unfairly flagged as “Partly False Information.”
I was trying to post on IG that folks should not put up the Tuesday tag alongside the BLM tag and I just grabbed an image and reposted. And then it got flagged in 15 min. #algorithmsofoppression pic.twitter.com/dkALdH2fG9
— Safiya Umoja Noble PhD (@safiyanoble) June 3, 2020
As a result, labels should offer swift recourse to contest labels and provide feedback if users feel labels have been inappropriately applied, similar to interactions for reporting harmful posts.
Additionally, platforms should share the reasoning and process behind the label’s application. If an instance of manipulated media was identified and labeled through automation, for example, without context-specific vetting by humans, that should be made clear to users. This also means linking the label to an explanation that not only describes how the media was manipulated, but who made that determination and should be held accountable. Facebook’s labels, for example, will say that the manipulated media was identified by third-party fact-checkers, and clicking the “See Why” button opens up a preview of the actual fact check.
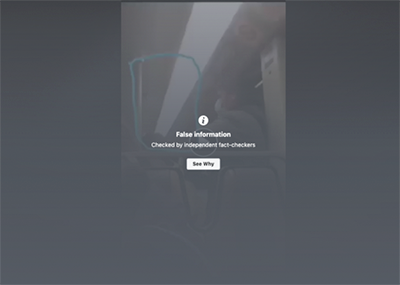
Example of a Chinese-language video fact-checked and flagged as containing false information related to coronavirus.
10. Fill in missing alternatives with multiple visual perspectives
Experiments have shown that a debunk is more effective at dislodging incorrect information from a person’s mind when it provides an alternative explanation to take the place of the faulty information [3]. Labels for manipulated media should present alternatives that preempt the user’s inevitable question: what really happened then?
Recall that the “picture superiority effect” means images are more likely to be remembered than words. If possible, fight the misleading visuals with accurate visuals. This can be done by displaying multiple photo and video perspectives to visually reinforce the accurate event [5]. Platforms could also explore displaying “related images,” for example by surfacing reverse image search results, to provide visually similar images and videos possibly from the same event.
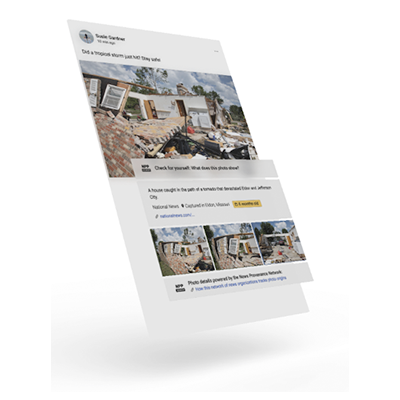
Prototype featuring multiple images for the News Provenance Project, a New York Times R&D initiative
11. Help users identify and understand specific manipulation tactics
Research shows that people are poor at identifying manipulations in photos and videos: In one study measuring perception of photos doctored in various ways, such as airbrushing and perspective distortion, people could only identify 60 per cent of images as manipulated. They were even worse at telling where exactly a photo was edited, accurately locating the alterations only 45 per cent of the time [18].
Even side-by-side presentation of manipulated and original visuals may not be enough without extra indications of what has been edited,. This is due to “change blindness,” a phenomenon where people fail to notice major changes to visual stimuli: “It often requires a large number of alternations between the two images before the change can be identified…[which] persists when the original and changed images are shown side by side” [19].
These findings indicate that manipulations need to be highlighted in a way that is easy to process in comparison to any available accurate visuals [18], [19]. Showing manipulations beside an unmanipulated original may be useful especially when accompanied by annotated sections of where an image or video has been edited, and including explanations of manipulations to aid future recognition.
In general, platforms should provide specific corrections, emphasizing the factual information in close proximity to where the manipulations occur — for example, highlighting alterations in context of the specific, relevant points of the video [20]. Or, if a video clip has been taken out of context, show how. For example, when an edited video of Bloomberg’s Democratic debate performance was misleadingly cut together with long pauses and cricket audio to indicate that his opponents were left speechless, platforms could have provided access to the original unedited debate clip and describe how and where the video was edited to increase user awareness of this tactic.
12. Adapt and measure labels according to the use case
In addition to individual user variances, platforms should consider use case variances: interventions for YouTube, where users may encounter manipulated media through searching specific topics or through the auto-play feature, might look different from an intervention on Instagram for users encountering media through exploring tags, or an intervention on TikTok, where users passively scroll through videos. Before designing, it is critical to understand how the user might land on manipulated media, and what actions the user should take as a result of the intervention.
Is the goal to have the user click through to more information about the context of the media? Is it to prevent users from accessing the original media (which Facebook has cited as its own metric for success)? Are you trying to reduce exposure, engagement, or sharing? Are you trying to prompt searches for facts on other platforms? Are you trying to educate the user about manipulation techniques?
Finally, labels do not exist in isolation. Platforms should consider how labels interact with other contexts and credibility indicators around a post (eg social cues, source verification). Clarity about the use and goals are crucial to designing meaningful interventions.
These principles are just a starting point
While these principles can serve as a starting point, they are no replacement for continued and rigorous user-experience testing across diverse populations, tested in actual contexts of use. Much of this research was conducted in artificial contexts that are not ecologically valid, and as this is a nascent research area, some principles have been demonstrated more robustly than others.
Given this, there are inevitable tensions and contradictions in the research literature around interventions. Ultimately, every context is different: abstract principles can get you to an informed concept or hypothesis, but the design details of specific implementations will surely offer new insights and opportunities for iteration toward the goals of reducing exposure to and engagement with mis/disinformation.
To move forward in understanding the effects of labeling requires that platforms publicly commit to the goals and intended effects of their design choices; and share their findings against these goals so as to be held accountable. For this reason, PAI and First Draft are collaborating with researchers and partners in industry to explore the costs and benefits of labeling in upcoming user experience research of manipulated media labels.
Stay tuned for more insights into best practices around labeling (or not labeling) manipulated media, and get in touch to help us thoughtfully address this interdisciplinary wicked problem space together.
If you want to speak to us about this work, email contact@firsrtdraftnews.com or direct message us on Twitter. You can stay up to date with all of First Draft’s work by becoming a subscriber and following us on Facebook and Twitter.
Citations
- Swire, Briony, and Ullrich KH Ecker. “Misinformation and its correction: Cognitive mechanisms and recommendations for mass communication.” Misinformation and mass audiences (2018): 195–211.
- The Legal, Ethical, and Efficacy Dimensions of Managing Synthetic and Manipulated Media https://carnegieendowment.org/2019/11/15/legal-ethical-and-efficacy-dimensions-of-managing-synthetic-and-manipulated-media-pub-80439
- Cook, John, and Stephan Lewandowsky. The debunking handbook. Nundah, Queensland: Sevloid Art, 2011.
- Infodemic: Half-Truths, Lies, and Critical Information in a Time of Pandemics https://www.aspeninstitute.org/events/infodemic-half-truths-lies-and-critical-information-in-a-time-of-pandemics/
- The News Provenance Project, 2020 https://www.newsprovenanceproject.com/
- Avram, Mihai, et al. “Exposure to Social Engagement Metrics Increases Vulnerability to Misinformation.” arXiv preprint arXiv:2005.04682 (2020).
- Bago, Bence, David G. Rand, and Gordon Pennycook. “Fake news, fast and slow: Deliberation reduces belief in false (but not true) news headlines.” Journal of experimental psychology: general (2020).
- Pennycook, Gordon, and David G. Rand. “Lazy, not biased: Susceptibility to partisan fake news is better explained by lack of reasoning than by motivated reasoning.” Cognition 188 (2019): 39–50.
- Lutzke, L., Drummond, C., Slovic, P., & Árvai, J. (2019). Priming critical thinking: Simple interventions limit the influence of fake news about climate change on Facebook. Global Environmental Change, 58, 101964.
- Karduni, Alireza, et al. “Vulnerable to misinformation? Verifi!.” Proceedings of the 24th International Conference on Intelligent User Interfaces. 2019.
- Metzger, Miriam J. “Understanding credibility across disciplinary boundaries.” Proceedings of the 4th workshop on Information credibility. 2010.
- Nyhan, Brendan. Misinformation and fact-checking: Research findings from social science. New America Foundation, 2012.
- Wood, Thomas, and Ethan Porter. “The elusive backfire effect: Mass attitudes’ steadfast factual adherence.” Political Behavior 41.1 (2019): 135–163.
- Nyhan, Brendan, and Jason Reifler. “When corrections fail: The persistence of political misperceptions.” Political Behavior 32.2 (2010): 303–330.
- Clayton, Katherine, et al. “Real solutions for fake news? Measuring the effectiveness of general warnings and fact-check tags in reducing belief in false stories on social media.” Political Behavior (2019): 1–23.
- Badrinathan, Sumitra, Simon Chauchard, and D. J. Flynn. “I Don’t Think That’s True, Bro!.”
- Ticks or It Didn’t Happen: Confronting Key Dilemmas In Authenticity Infrastructure For Multimedia, WITNESS (2019) https://lab.witness.org/ticks-or-it-didnt-happen/
- Nightingale, Sophie J., Kimberley A. Wade, and Derrick G. Watson. “Can people identify original and manipulated photos of real-world scenes?.” Cognitive research: principles and implications 2.1 (2017): 30.
- Shen, Cuihua, et al. “Fake images: The effects of source, intermediary, and digital media literacy on contextual assessment of image credibility online.” new media & society 21.2 (2019): 438–463.
- Diakopoulos, Nicholas, and Irfan Essa. “Modulating video credibility via visualization of quality evaluations.” Proceedings of the 4th workshop on Information credibility. 2010.